Mike Laszkiewicz
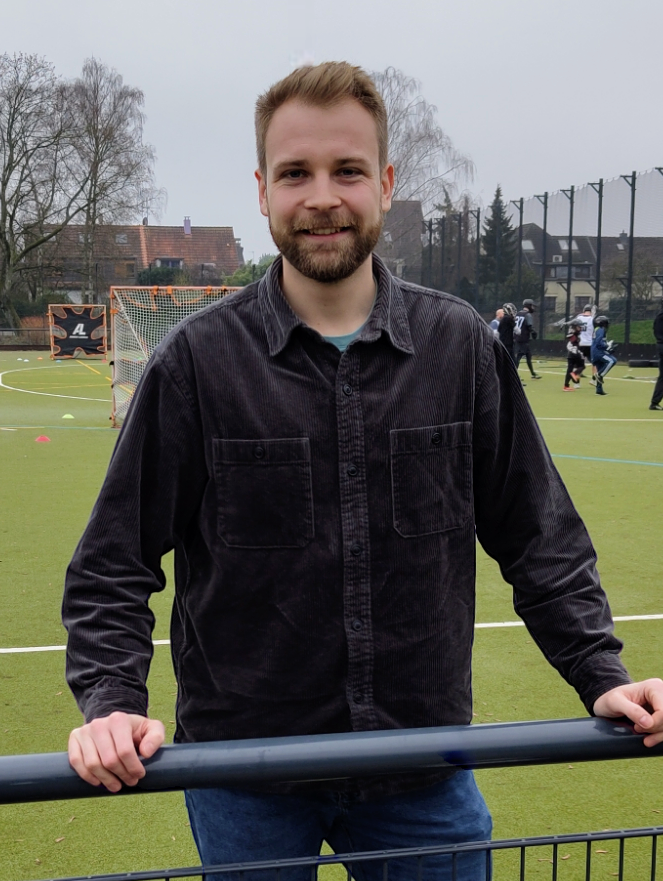
Doctoral student working in the Machine Learning group at RUB. My research revolves around deep generative models, such as generative adversarial networks, normalizing flows, diffusion models, and more. I seek to understand those models, uncover their weaknesses, and implement practical improvements, which broaden their fields of application.
In my spare time, I like to work on fun programming projects and improve my skills for my other passion: playing Lacrosse!
News
May 3, 2024 | Exciting news! Our paper, “Single-Model Attribution of Generative Models Through Final-Layer Inversion”, got accepted at ICML 2024 in Vienna! In this work, we address model attribution of generative models by leveraging modern anomaly detection techniques. To improve the performance further, we combine the latter with features extracted by final-layer inversion. Please find our preprint on arXiv. Thanks for the collaboration, Jonas! |
---|---|
Apr 10, 2024 | I am happy to share that our paper, “Benchmarking the Fairness of Image Upsampling Methods”, has been accepted at ACM FAccT 2024. 🇧🇷 In this work, we introduce a comprehensive framework for benchmarking the performance and fairness of conditional generative models. We explore the specific application of image upsampling and create a benchmark covering a wide variety of modern upsampling methods. Check out our preprint on arXiv. Thanks to my awesome collaborators at ETH Zurich, UHH, and RUB! |
Sep 20, 2023 | ![]() ![]() Besides giving a talk about my current project on Fairness of Image Upsampling Methods, I was delighted to discuss further ideas about fair generative modeling with Prof. Mohamed Hebiri and his team from the Université Gustave Eiffel. Thanks for having me! |
Jul 1, 2023 | Exciting Announcement: Embarking on a Research Adventure at ETH Zurich’s Medical Data Science Group! Over the next two months, I will have the privilege to work on the development and enhancement of fairness in deep conditional generative models at ETH. |
Apr 25, 2023 | I got the opportunity to give a short presentation of my research on theory of generative modeling at the Postdoctoral Networking Tour in Artificial Intelligence (Postdoc-NeT-AI), a DAAD initiative funded by the Federal Ministry of Education and Research. |